2017年5月11日(星期四)下午14:50,第289期同路人学术论坛在通达馆103室顺利举行。本次论坛由马万经教授主持,邀请来了孙立君博士、许岩岩博士、曾伟良博士三位优秀青年学者,为大家带来主题为“Traffic Analysis and Design”的讲座。
(1) Optimal Design of Urban Traffic Intersections
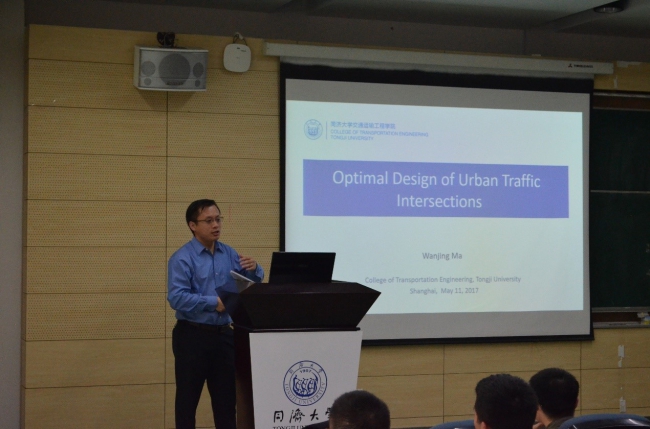
马万经教授主要研究交通设计与控制、共享交通、车路协同和智能交通系统等。在Transportation Research Part B和等国内外核心期刊和学术会议发表论文100余篇。先后主持完成国家自然科学基金2项,省部级科研课题5项,参与国家自然科学基金重点项目、国家863计划课题、科技支撑计划等理论研究和基础应用课题10余项。参与编写专著3部,参加翻译规范 1部,参编国家十一五、十二五规划教材2部;参编国家和行业规范标准3部。
先后获评霍英东青年教师基金(2016)、上海市浦江人才计划(2014)、36365线路检测中心no1青年教学科研骨干计划(2013)、36365线路检测中心no1青年岗位能手(2011)、36365线路检测中心no1优秀青年教师(2010),36365线路检测中心no1青年教师讲课竞赛一等奖(第一名,2009)。研究成果先后获得中国智能交通协会科学技术奖一等奖1项,上海市科技进步二等奖1项,三等奖1项,建设部华夏建设科技奖1项,山东省优秀城市规划设计奖1项,被评为Transportation Research Part C 杰出审稿人(2015)。
目前兼任上海市交通委科学技术委员会委员,上海交通工程学会会员,人工智能学会智能交通专业委员会委员,中国自动化学会综合智能交通专业委员会。是交通领域顶级期刊Transportation Research Part C 等两个国际期刊编委,Transportation Research Board (ANB80T)委员,World Conference on Transport Research Society (SG3)委员,20多个国内国际期刊的审稿人。
(2) Harnessing Data Analytics in Urban Public Transport Operation and Planning
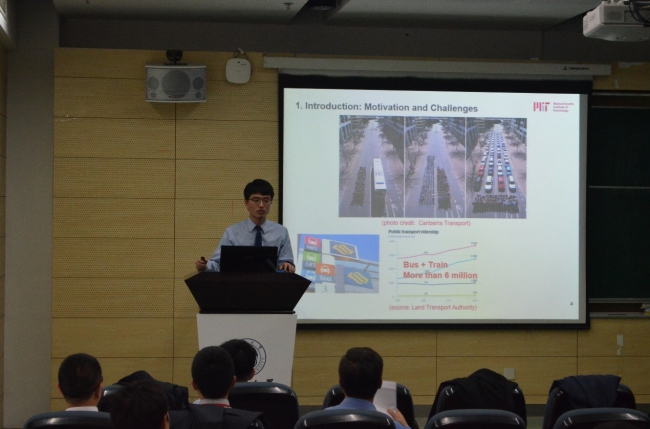
Lijun Sun is a Postdoctoral Associate at MIT Media Lab (https://www.media.mit.edu/). His current research focuses on developing and applying crowd sourcing and data-driven approaches in the domain of civil systems and transportation. He earned his B.S. degree in Civil Engineering from Tsinghua University in 2011 and his Ph.D. degree in Transportation from National University of Singapore in 2015. Before joining MIT, he worked at Future Cities Laboratory (http://www.fcl.ethz.ch/), Singapore-ETH Centre as a PhD researcher and then a senior research fellow in the Mobility and Transportation Planning Module, combining smart card-driven public transport modeling and agent-based simulation to improve urban public transport service quality and reliability.
In recent years, the emergence of massive individual-based datasets and advances in informatics and data science have transformed our understanding in a variety of fields in transportation research. It also motivates a new way of data-driven transport approach through conducting extensive analyses and building realistic models. For example, behavior pattern inference from spatial-temporal data set has facilitated the development of urban public transport in both day-to-day operation and long-term planning. However, in a large-scale and highly-congested city, the application of these technologies remains prone to operational pitfalls and obstacles. In this talk, he presents about harnessing various data analytics and computational models to tackle resilience issues in public transport operation and planning, by integrating machine learning, operations research and behavioral economics. He mainly discussed a typical topic about passenger behavior inference to show the basic concept of combining data analytics and computational models, and illustrate how it can be used to improve the resilience of public transport systems. The presented methodologies can be integrated with an agent-based modeling/simulation framework, and further help plan and evaluate future transportation systems (e.g., on-demand mobility services) for “resilient smart cities”.
(3) Big Data Driven Mobility to Tackle Urban Traffic and Electric Vehicle Management
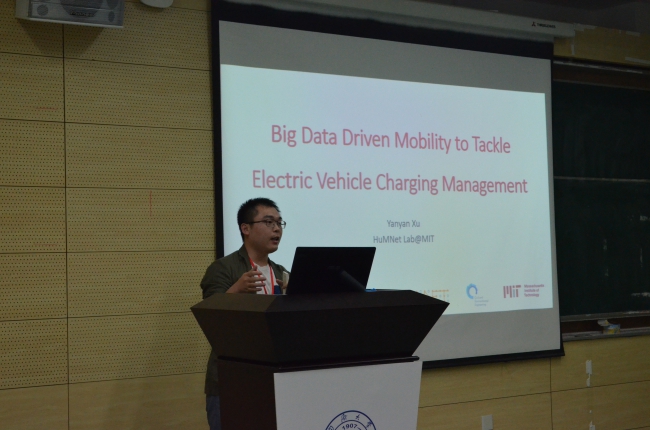
Yanyan Xu is a postdoctoral associate in the Human Mobility and Networks Lab, Department of Civil and Environmental Engineering, MIT. He received PhD in Pattern Recognition and Intelligent Systems from Shanghai Jiao Tong University, China, in 2015. He works in the fields of data mining, human mobility, with a focus on the use of information and communication technology and big data in Transportation Systems, Environment, and Urban Planning. Specific projects include urban traffic flow prediction, travel demand management using big data; revealing the impacts of traffic on the air quality using mobile phone data; and coupling electric vehicle charging with urban mobility, etc. His work has been published in the J. Roy. Soc. Interface, IEEE Trans. ITS, J. Adv. Transp., TRB, among others.
Understanding human mobility has many applications in diverse areas, including spread of diseases, city planning, traffic engineering, financial market forecasting, and nowcasting of economic well-being. In the past years, we have studied problems concerning the use of various sources of large-scale data to better inform human mobility and collective travel behavior in cities. In this presentation, he introduced two main works on the applications of human mobility: traffic demand management and the Electric Vehicles charging planning. In the first task, our target is to understand the impact of mega events using multiple data resources and design feasible travel demand management strategy for a global mitigation of traffic congestion. In the second one, we couple the urban mobility with EV charging plan to alleviate the pressure of power grid from the EV charging. In detail, we represent the mobility of EV drivers in San Francisco Bay Area in USA, and shift the arrival and departure times of EV drivers to reduce the peak load of EV energy demand in the working area.
(4) Exploring traveler's risk preference to travel time uncertainty from large-scale GPS trip data
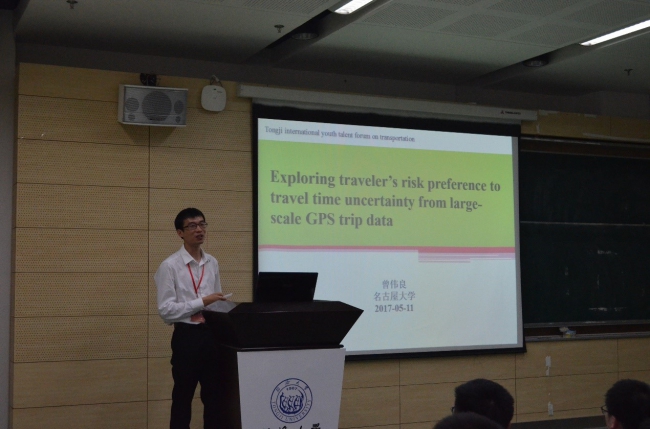
Dr. Weiliang Zeng is a postdoctoral researcher in Institution of Materials and Systems for Sustainability, Nagoya University, Japan. He received the B.S. and M.S. degrees from the College of Engineering, Sun Yat-sen University in 2009 and 2012, respectively. In 2012, he came to Nagoya University for further study, and received PhD degree in 2016.His current research interests include reliable routing problem, autonomous taxi system, pedestrian simulation etc. He received the best paper award in Eastern Asia Society for Transportation Studies (EASTS) in 2015. He is also the reviewer for TRC, TRE, IEEE ITS, Chinese Physics B,and Urban Rail Transit.
Travel time reliability has found notable interest in route choice modelling. Knowing how individuals choose paths with uncertain travel times is fundamental to advance our understanding of route choice behavior and drive the development of route guidance systems. Current navigation systems usually provide shortest paths based on distance or travel time, despite many travelers do not choose the shortest one. Many studies pointed out that the probability of delay or travel time reliability is an important factor in traveler’s route choice decision. Traditionally, route choice data for individual preference analysis can be collected by stated preference surveys. However, it requires repeated interaction with the travelers, and inherent limitations related to honest, accurate and bias-free reporting are difficult to avoid. To fill this gap, this study proposes a new data collection methodology that enables to estimate traveler’s risk-averse preference by GPS trip record. The lower bound and upper bound of individual risk-averse preference can be estimated by exhausting a series of reliable paths with different on-time arrival probabilities and using the theory of stochastic dominance. Then, a regression model based on logistic function is established to explore how the influencing factors impact on the lower and upper bounds of risk-averse preference. It is found that the individual properties such as age and pre-trip information such OD distance, departure time, and day of week are significant to the degree of risk-averse preference.
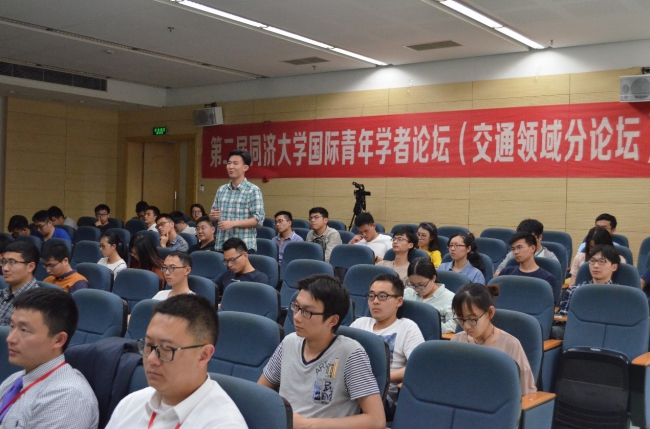
在每一部分介绍后,会留有5分钟时间用于与会师生的交流讨论,现场互动热烈,通过对交通领域新技术、新方法的探讨与交流,每个人深受启发。